Embracing Predictive Analytics for Demand Forecasting in Logistics
Companies striving to stay competitive must effectively predict customer demand, optimize their operations, and ensure efficient inventory management. However, accurate demand forecast is not an easy task, given the complexities and uncertainties inherent in logistics operations.
Challenges
Logistics companies face numerous challenges when it comes to demand forecasting. These challenges arise due to various factors such as rapidly changing market conditions, seasonality, complex product portfolios, and evolving customer preferences. Traditional methods of demand forecasting, such as historical data analysis and expert opinions, often fall short in accurately capturing the dynamic nature of demand patterns.
Demand volatility
One of the major challenges is the presence of demand volatility, where demand patterns fluctuate frequently. Seasonal products, promotional campaigns, and unpredictable external factors contribute to this volatility, making it difficult for logistics companies to accurately predict future demand.
Demand fragmentation
Logistics companies often deal with a wide range of products across different categories, each exhibiting unique demand patterns. It becomes essential to analyze these demand patterns at a granular level to make accurate forecasts and optimize operations accordingly.
Embracing Predictive Analytics for Demand Forecasting in Logistics
Predictive analytics leverages advanced statistical modeling techniques, machine learning algorithms, and real-time data analysis to forecast future demand accurately.
By harnessing the power of predictive analytics, logistics companies can analyze vast quantities of historical and real-time data to identify trends, patterns, and correlations. This allows them to make more informed predictions about future demand, considering various factors such as seasonality, promotions, historical sales, and external events.
Predictive Analytics
Predictive analytics is a powerful tool used in demand forecasting in the logistics industry. It involves the use of various techniques, such as machine learning and data mining, to uncover patterns and insights from historical data to make accurate predictions about future demand.
Machine learning
This approach involves building mathematical models and algorithms that can learn from historical data to make predictions or take actions without being explicitly programmed. Machine learning algorithms analyze large sets of historical data, identify patterns, and use these patterns to predict future demand. By continuously learning from new data, these algorithms can adapt and improve their accuracy over time.
Data mining
It involves the process of discovering patterns, correlations, and trends within large datasets. In demand forecasting, data mining techniques are used to extract valuable insights from historical sales data, customer behavior, market trends, and other relevant information. These insights can then be used to create accurate demand forecasts.
Combination
The combination of machine learning and data mining allows logistics companies to leverage vast amounts of data to uncover hidden factors that influence demand. For example, they can identify seasonal trends, external factors like weather conditions or economic indicators, and even customer preferences that impact product demand. By understanding these patterns and factors, logistics companies can proactively adjust their inventory levels, allocate resources efficiently, and optimize their operations to meet changing customer demands.
Benefits of Embracing Predictive Analytics in Logistics
By embracing this powerful tool for demand forecasting, logistics companies can unlock a myriad of benefits that can revolutionize their operations.
Improving accuracy of demand forecasting
Traditional methods often rely on historical data and basic mathematical formulas, which may not accurately capture the complex dynamics of today’s supply chains. Predictive models, on the other hand, consider a wide range of variables such as trends, seasonality, market conditions, and even external factors like weather patterns. By incorporating these factors, predictive models can provide more accurate and reliable demand forecasts, helping logistics companies make informed and strategic decisions.
Enhancing efficiency in logistics operations
With accurate demand forecasts at their disposal, companies can optimize their transportation, warehousing, and inventory management processes. For instance, knowing the expected demand for a particular product in a specific region can help logistics companies plan their shipments more effectively, reducing transportation costs and minimizing lead times. Moreover, accurate predictions enable companies to allocate their resources efficiently, ensuring that customer orders are fulfilled promptly, leading to improved customer satisfaction.
Better inventory management
One of the challenges faced by logistics companies is striking the right balance between having enough stock to meet customer demand and avoiding excess inventory. Predictive analytics allows for proactive inventory management by analyzing historical data, market trends, and other relevant factors. With this information, logistics companies can determine optimal stock levels, ensuring they have sufficient inventory to meet demand while minimizing the risk of stockouts or overstocking. This not only leads to cost savings but also eliminates the potential loss of sales due to stockouts or the unnecessary tying up of capital in excess inventory.
Implementing Predictive Analytics in Demand Forecasting
Implementing predictive analytics involves several steps that ensure the effectiveness and reliability of the forecasting models:
Data collection and preprocessing
This includes historical sales data, customer information, market trends, and any other sources that can provide insights into demand patterns. The quality of the data is of utmost importance, as inaccurate or incomplete data can lead to flawed forecasting models.
Once the data is collected, it needs to be preprocessed. This involves cleaning the data by removing any outliers, duplications, or errors. Data normalization techniques are also applied to ensure consistency and comparability across different variables. Preprocessing the data is crucial to ensure the accuracy and reliability of the predictive models.
Model development
After the data has been collected and preprocessed, the next step is to develop predictive models using machine learning algorithms. These algorithms analyze the historical data to identify patterns and relationships that can be used to predict future demand. Various machine learning techniques, such as regression analysis, time-series analysis, and neural networks, can be applied depending on the nature of the data and the forecasting requirements.
During the model development phase, companies need to select the most appropriate algorithms and fine-tune the model parameters to enhance accuracy. This often requires iterative testing and refinement to ensure optimal performance. It is important to involve domain experts and data scientists during this process to leverage their expertise and ensure the models align with business objectives.
Model evaluation and validation
Once the predictive models have been developed, they need to be evaluated and validated to assess their accuracy and reliability. This entails comparing the forecasts generated by the models with actual demand data. Metrics such as mean absolute percentage error (MAPE) and root mean square error (RMSE) are commonly used to measure the accuracy of the forecasts.
The validation process helps identify any limitations or shortcomings in the models and provides an opportunity for further improvement. It is crucial to continuously monitor and validate the models as market dynamics change over time.
Integration and deployment
The final step in implementing predictive analytics for demand forecasting is integrating the models into existing logistics systems. The predictive models can be integrated into enterprise resource planning (ERP) systems, transportation management systems (TMS), or warehouse management systems (WMS) to enable real-time demand forecasting.
Integration allows companies to leverage the forecasting insights to make informed decisions regarding inventory management, production planning, and transportation scheduling. It enables proactive response to changing customer demands, reduces stock-outs, and minimizes excess inventory. Seamless integration also ensures the availability of accurate and up-to-date demand forecasts throughout the supply chain, optimizing overall logistics operations.
Challenges
Data quality and availability
Accurate predictions require good data from different sources: historical sales, customer behavior, and market trends. But organizations struggle to collect and maintain this data. It’s often scattered across different systems and departments.
To tackle this challenge, logistics companies must invest in data management systems and processes. These systems should be capable of handling large amounts of data, ensuring its accuracy, and integrating data from various sources. This includes data cleansing and validation techniques to remove duplicates, errors, and inconsistencies. Furthermore, organizations must establish protocols to ensure consistent data collection and storage practices to maintain data integrity and reliability.
Organizational readiness
Embracing predictive analytics requires a cultural shift within the organization, as well as change management efforts to ensure successful adoption. This involves creating awareness and buy-in from stakeholders at all levels, including executives, managers, and frontline employees.
Organizational readiness entails providing adequate training and resources to employees and developing a clear roadmap for implementation. It also involves aligning roles and responsibilities to leverage predictive analytics effectively and integrating it into existing processes and workflows. Leadership support and effective communication are essential for organizational readiness. They aid in overcoming resistance to change and promote a positive and collaborative atmosphere.
Future Trends and Conclusion
In recent years, predictive analytics has gained significant traction in the field of logistics for demand forecasting. As technology continues to advance, there are several emerging trends and advancements that are expected to reshape the landscape of predictive analytics for demand forecasting in logistics.
AI
One major trend is the integration of artificial intelligence (AI) and machine learning algorithms into predictive analytics systems. These technologies have the ability to analyze vast amounts of data from various sources, such as customer behavior, market trends, and historical sales data, to generate more accurate and precise demand forecasts. By leveraging AI and machine learning, logistics companies can make more informed decisions about inventory management, supply chain optimization, and resource allocation.
Real-time data + IoT
Another promising trend is the use of real-time data and IoT devices. Logistics companies have access to real-time data from IoT devices and sensors. This data includes information on factors that can affect demand, like weather conditions, traffic congestion, and product popularity. By using this real-time data in predictive analytics models, logistics companies can promptly adapt to changes in demand. This helps them avoid running out of stock or having excess inventory.
Cloud computing and big data analytics
Cloud-based predictive analytics platforms offer scalability and flexibility. This allows logistics companies to store and analyze large volumes of data. They can do this without the need for extensive IT infrastructures. This reduces the barriers to entry and enables smaller companies to embrace predictive analytics for demand forecasting.
Conclusion
In conclusion, using predictive analytics brings numerous benefits. These include improved inventory management, cost savings, and better customer satisfaction. The advantages outweigh any challenges that may arise. By taking advantage of the emerging trends and advancements in predictive analytics, logistics companies can gain a competitive edge. They can also ensure that their supply chain operations are efficient and effective.
Invitation to free consultation
We are inviting you to a free consultation with Signalo. Signalo is an experienced creator of various systems for international corporations. These systems have successfully streamlined processes and reduced costs for many companies. The purpose of the free consultation is to assess your specific needs. We will provide a tailored proposal for custom software solutions in the area you want to improve.
Manufacturing software
IT Systems
What challenge would you like to tackle?
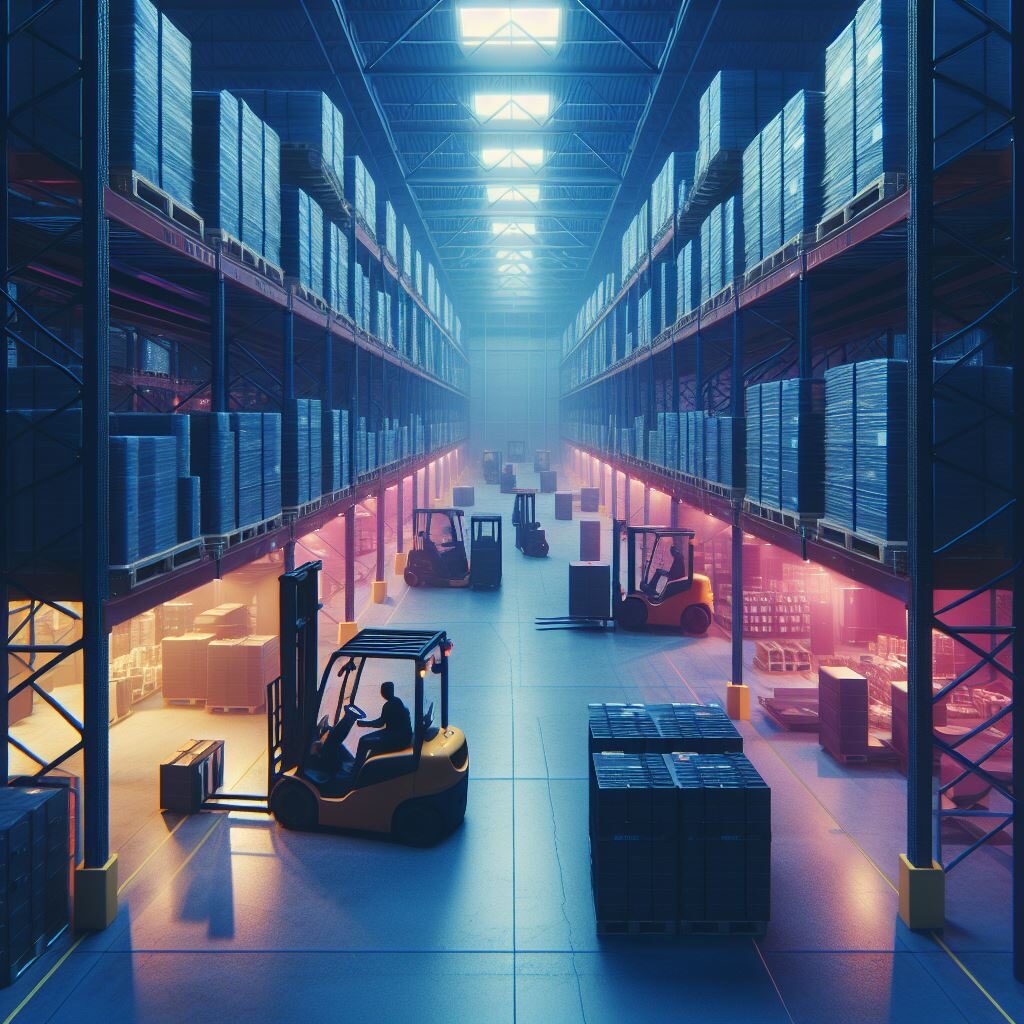
- Optimization of internal logistics
- Recalls of production sockets
- Messages from machines
- Monitoring electricity, water, gas, temperature, humidity, and vibrations
- Streamlining production
- Management and control of tool distribution
- Managing employee skills
- Collecting employee ideas for improvements
- Digital safety workplace instructions
- Improving safety
Values
Discover the benefits of collaboration
We offer a free consultation as the first step of collaboration, allowing you to familiarize yourself with our approach and verify our advisory competencies. This way, even before making a decision, you’ll feel that your situation and problem have been well understood, and the proposed solution will be optimally tailored to your company’s situation.
Our services (support) are included in a subscription, which does not generate hidden costs. The subscription-based billing allows for a significant reduction in the entry threshold into the system and enables immediate testing.
We are communicationally independent – we can develop our system without depending on the client’s IT department. We do not generate additional work for the client’s IT department. This way, we lower the implementation risk and associated costs. The possibility of quicker implementation of the solution results in faster return on investment.
Login to our applications is secured at a high level, characteristic of banking solutions. At the same time, the login process is intuitive. Your data is as secure as in a bank thanks to our system.
We offer multilingualism in our solutions through real-time translation—each employee operates applications in their native language. This enhances efficiency and reduces costs by expanding the potential labor market and eliminating the need to hire translators.
Our software features an interface optimized for quick, easy, and intuitive use, even by digitally excluded or poorly skilled employees. This means real-time savings in both time and training costs for your workforce in using the new software. The ability for rapid deployment of software for use results in a shorter transition/implementation period.
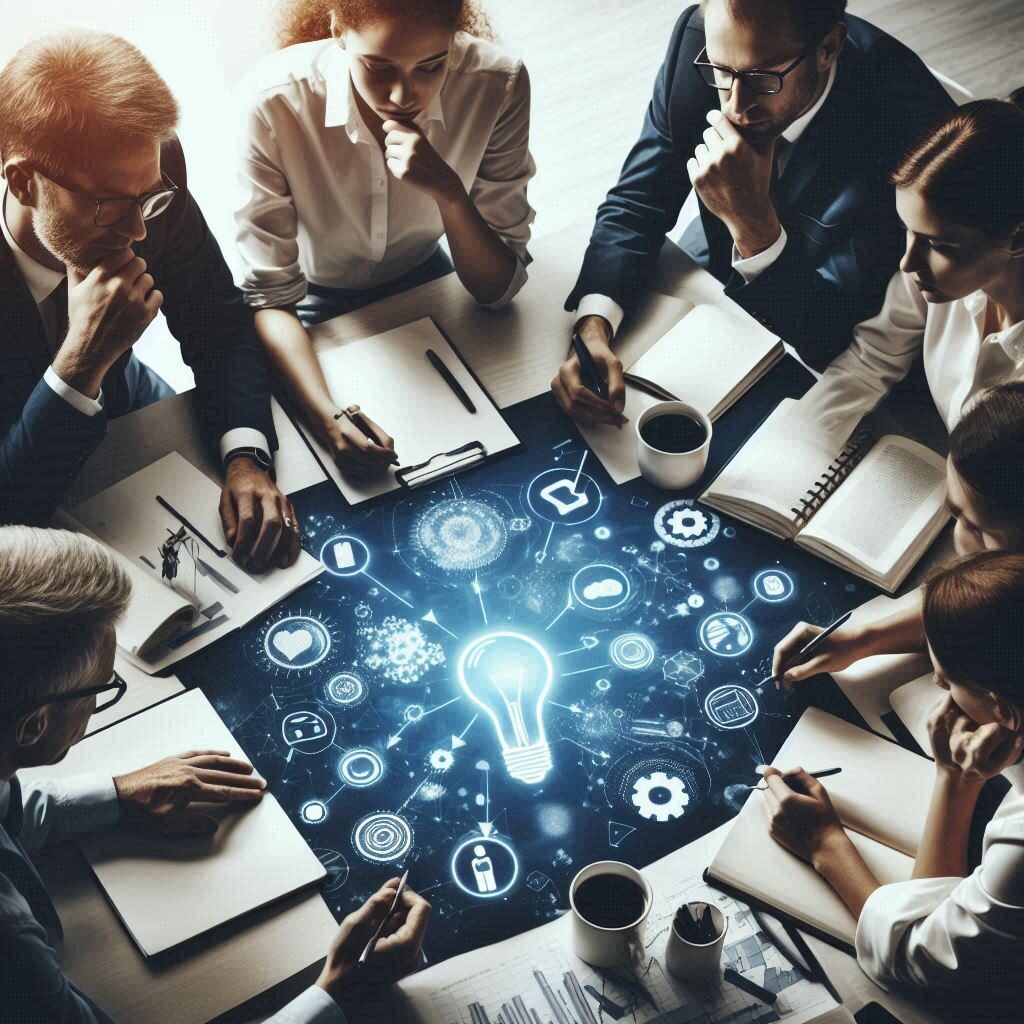